Compare International Money Transfers
Our Free Price Comparison Services
We show you how to pay less for foreign currency
Compare rates from all the major foreign exchange providers in one place. Simply choose how you want to exchange money to get started:
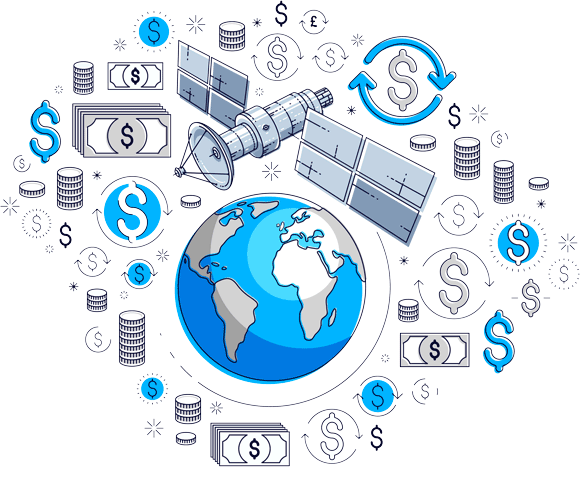
Cheap
Find the cheapest currency provider from our carefully-vetted panel of dozens, using our free search tools. You will never pay extra commission for being introduced by us.
Reliable
Read genuine, uncensored reviews of currency providers, from real users like you. Crowd feedback keeps only the most reliable currency providers listed here.
Secure
This site, and every site we link to, runs on Secure Socket Layer technology and adheres to all data protection laws, to keep your details from falling into the wrong hands.
Informed
Browse our knowledge base in the resources section and our blog. The articles are full of tips to avoid getting ripped off when buying or selling currency online.